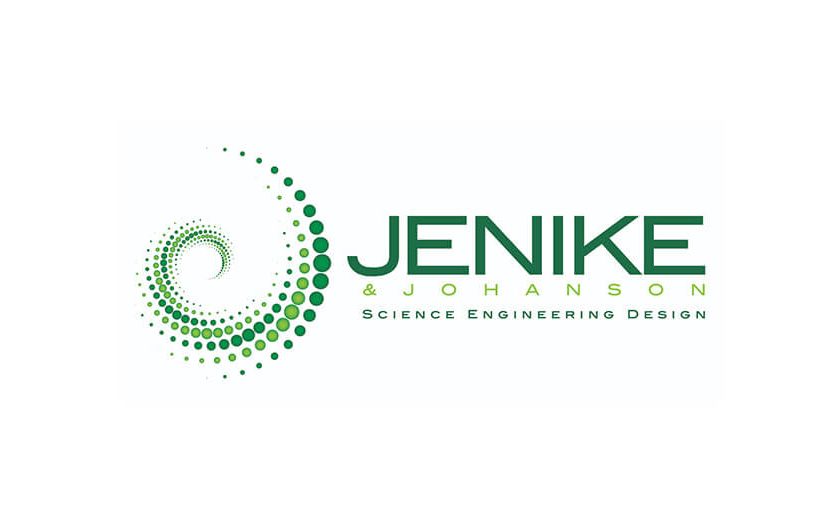
I recently worked on a project where one of the biggest objectives was improving the uniformity of the bulk material feed rate to the downstream process. The fine powder material was metered by a loss-in-weight feeder, along a conveyor, and into a downstream process. Due to some issues with the loss-in-weight bin and feeder design, the feeders were often subject to large rate variations. Additionally, the slow speed conveyor tended to induce rate variation as well, as the material would slough in rhythm to each conveyor flight arrival.
Unfortunately, completely replacing the loss-in-weight feeders was not a feasible option for the client due to facility and budgetary constraints. As an alternative, we proposed a new fluidized surge bin between the existing conveyors and the downstream process. The fluidized surge bin would act as a damper between the existing feeders and conveyors and the downstream process.
Our initial material tests demonstrated the feasibility of this concept. Nonetheless, there were still many questions about how this bin would perform at full scale to damp the levels of rate variation from the upstream system. As a confounding factor, the discharge rate from a fluidized bin is dependent on the head of material in the bin.
To analyze the prospective performance of the new bin, we created a computer model of the client’s entire process, including the existing loss-in-weight feeders, conveyors, and the proposed new bin. As an input to the model, our client was able to provide output rate data from the loss-in-weight feeders over the course of two hours. Our model tracked the material that was output from the loss-in-weight feeders through the conveyors and into the fluidized surge bin.
We were able to show that for a wide range of operating conditions at the loss-in-weight feeders, the level fluctuations were relatively minor, and the rate uniformity could be expected to improve by at least an order of magnitude by the agreed metric. We were also able to show that rate variations due to sloughing in rhythm with the conveyor flights (which was of an unknown magnitude but thought to be occurring) would be virtually eliminated. Regardless of the sloughing magnitude, the model showed these rhythmic variations would be virtually eliminated due to their short period.
Of course, any computer model is only as good as the inputs to the model. For modeling systems with bulk materials, this is where understanding the properties of the material is critical; only then is it possible to create a meaningful model. From there, the possibilities for using the computer process model as a tool are vast. For instance, a process model could be used to help troubleshoot a complex process with multiple components and control systems. Changes to control logic or process steps can be considered in simulation prior to deploying them on the actual system. This can be helpful both as decision making tool and as a tool to dry run new process concepts before implementation. In either case, the key reason for a process model is ultimately saving both time and money. I look forward to working on more projects where we can help our clients with process models.